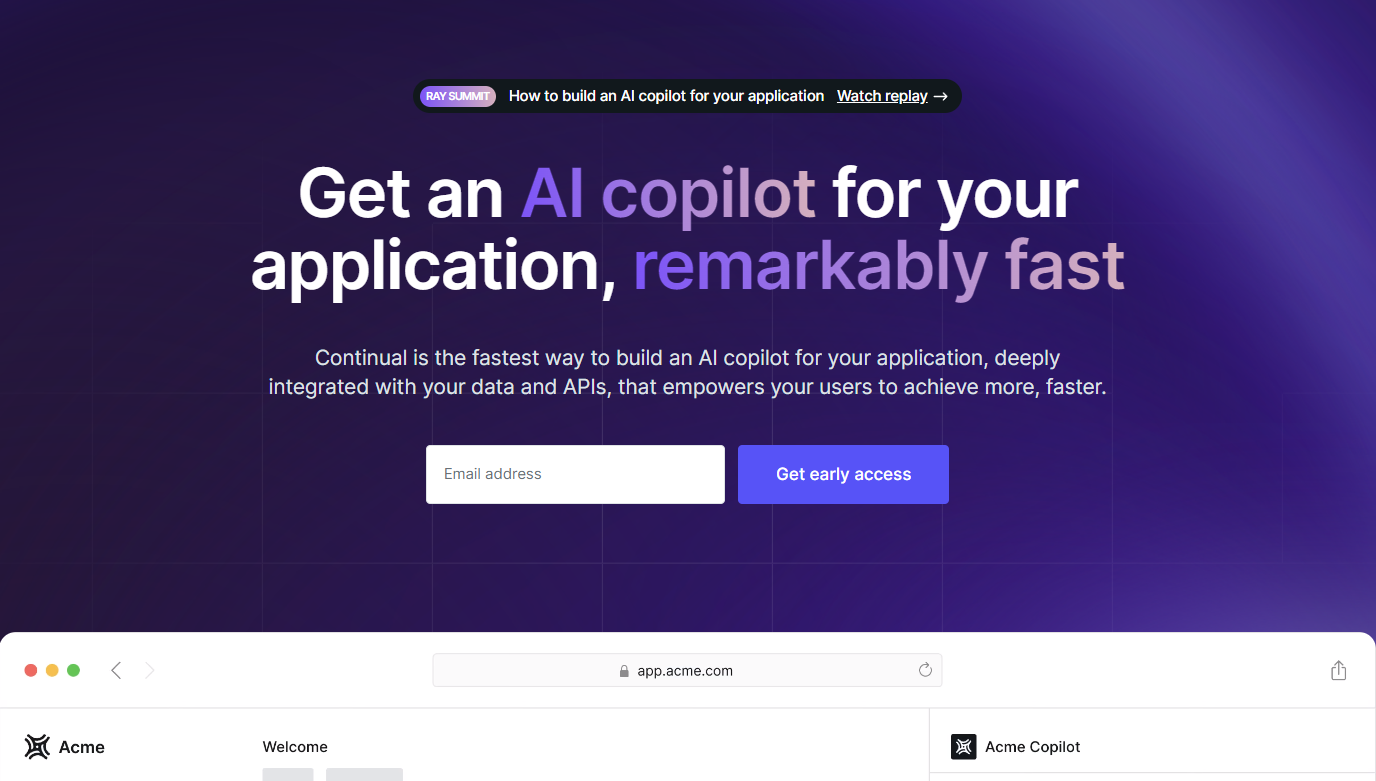
Embarking on the cutting edge of Artificial Intelligence, Continual AI surfaces as a transformative force, poised to revolutionize the very fabric of machine learning. This dynamic concept stands as a linchpin in the ever-evolving landscape of AI, holding the potential to redefine how machines adapt and grow. In the forthcoming exploration, we will unravel the layers of Continual AI, delving into its core principles and shedding light on the intricate facets that set it apart. This journey promises not just a glimpse but a comprehensive understanding of how Continual AI is reshaping the future of intelligent systems.
Table of Contents
Continual AI Overview
Definition of Continual AI
Continual AI, at its core, represents a revolutionary approach to machine learning that emphasizes continuous adaptation and learning over time. Unlike traditional models that often rely on static datasets, Continual AI thrives on a dynamic learning process, allowing machines to accumulate knowledge gradually, much like the way humans learn from diverse experiences. This shift in approach marks a departure from the static nature of conventional AI, promising a more fluid and adaptive system.
Differentiating Continual Learning vs Continuous Learning
It’s crucial to discern the distinction between Continual Learning and Continuous Learning within the context of AI. While Continuous Learning refers to a seamless, uninterrupted learning process without distinct phases, Continual Learning in AI involves adapting to new information while retaining knowledge from previous experiences. It’s akin to a continuous upgrade that builds upon existing knowledge, ensuring a more nuanced and versatile learning mechanism.
Unveiling the Theory and Principles behind Continual AI
The theoretical underpinnings of Continual AI are rooted in the concept of lifelong learning for machines. This approach acknowledges that learning is an ongoing process, and machines should adapt to new information without forgetting previously acquired knowledge. The principles involve creating flexible models that can assimilate new data seamlessly, preventing the “catastrophic forgetting” problem often encountered in traditional AI. Continual AI is built on the premise that learning should be a continuous, evolving journey, enabling machines to stay relevant in an ever-changing environment.
In this section, our exploration delves into the very essence of Continual AI. We dissect its definition, draw distinctions between Continual and Continuous Learning, and unveil the theoretical foundations that set the stage for its transformative role in the field of artificial intelligence.
Exploring Continual AI with ContinualAI
Overview of ContinualAI
At the heart of the Continual AI ecosystem lies its GitHub repository, a dynamic hub pulsating with innovation. Here, developers and AI enthusiasts converge to contribute, collaborate, and access a treasure trove of tools, frameworks, and models. ContinualAI’s GitHub repository serves as an open-source playground, fostering an environment where the principles of continual learning come to life through code. It encapsulates the collaborative spirit driving the evolution of Continual AI and serves as a testament to the community’s dedication to advancing the field.
Avalanche Continual AI: A Closer Look at Its Contributions
Within the ContinualAI universe, one stellar presence takes center stage—Avalanche Continual AI. This framework, intricately designed to tackle the challenges of continual learning, has left an indelible mark on the AI landscape. By dissecting its contributions, we uncover how Avalanche pioneers solutions to the notorious “catastrophic forgetting” problem. Its dynamic architecture enables machines to accumulate knowledge continually, preserving past learnings while seamlessly incorporating new insights. This deep dive into Avalanche reveals not just a tool but a groundbreaking contribution propelling Continual AI into new realms of effectiveness.
Navigating Through ContinualAI’s Presence on Crunchbase
The journey into ContinualAI extends beyond the realm of code to the concrete impact it has in the business world, a testament showcased on Crunchbase. Here, we navigate through the company’s profile, uncovering the strategic collaborations, funding, and industry partnerships that underscore ContinualAI’s significance. Crunchbase provides a panoramic view of how ContinualAI transcends theoretical realms, influencing the broader AI landscape with tangible results. It serves as a compass for investors, collaborators, and enthusiasts, guiding them through the real-world footprint of ContinualAI.
This section immerses readers into the practical dimensions of ContinualAI, exploring its GitHub repository as a nexus of innovation, unveiling the brilliance of Avalanche Continual AI, and navigating the tangible impact on Crunchbase.
Career Opportunities in Continual AI
A Glimpse into Continual AI Career Prospects
Embarking on a career in Continual AI unveils a landscape of unprecedented opportunities. As this revolutionary approach to machine learning gains traction, professionals find themselves at the forefront of a paradigm shift. From research and development roles to implementation and optimization specialists, the continuum of career prospects in Continual AI is vast. The industry beckons with a promise of continuous growth, echoing the very principles it operates on—ever-evolving and adaptive.
Skills and Expertise Sought in the Continual AI Domain
Within the dynamic realm of Continual AI, a unique set of skills and expertise emerges as the cornerstone of success. Adaptability takes precedence, mirroring the core principles of Continual Learning. Professionals navigating this domain must possess a keen understanding of machine learning algorithms, coupled with a mastery of programming languages like Python. Additionally, expertise in model deployment, data management, and a nuanced comprehension of the intricacies of continual learning frameworks, such as Avalanche Continual AI, become invaluable assets. The Continual AI domain prizes individuals who thrive in an environment of perpetual innovation and possess the ability to bridge theoretical knowledge with real-world applications.
In this section, we provide readers with a glimpse into the myriad career paths that unfold in the Continual AI realm. From the broader prospects to the specific skills coveted in this evolving landscape, the exploration aims to guide aspiring professionals toward a future where adaptability and continual learning are not just principles but the very fabric of success.
Unleashing the Potential: AI Improvement through Continual Learning
Insights from Research on AI Improvement
The journey into the realms of Continual AI extends beyond its applications to the broader landscape of AI improvement. Insights derived from extensive research underscore how Continual Learning has emerged as a catalyst for pushing the boundaries of artificial intelligence. Researchers delve into the nuances of continual learning frameworks, analyzing their impact on model performance, adaptability, and the mitigation of learning decay. These insights not only inform the continual enhancement of AI but also contribute to the evolution of the field itself.
Leveraging Continual Learning for Increasingly Accurate Predictions and Recommendations
At the heart of Continual AI’s prowess lies the ability to continually refine predictions and recommendations. By embracing the principles of continual learning, machines can adapt to dynamic datasets, evolving user preferences, and shifting contexts. The continuous accumulation of knowledge prevents stagnation, allowing AI systems to provide increasingly accurate insights over time. This approach stands in stark contrast to traditional models that may falter when faced with new information. Leveraging continual learning, AI transcends static predictions, ushering in a new era of adaptability, and precision.
In this segment, we delve into the transformative impact of Continual Learning on the broader AI landscape. Drawing insights from research, we uncover how continual learning frameworks contribute to the improvement of AI models and explore how this approach translates into increasingly accurate predictions and recommendations.
Diving Deeper: Continual AI Insights from Thought Leaders
Analyzing Key Content from the Continual AI LinkedIn Page
The pulse of Continual AI’s influence resonates on its LinkedIn page, where thought leaders and industry experts converge to share insights and updates. Analyzing key content provides a unique window into the forefront of Continual AI, revealing industry collaborations, success stories, and the latest advancements. Thought-provoking discussions unfold, offering perspectives on how Continual AI is shaping the future of machine learning. From career spotlights to technical deep-dives, the LinkedIn page serves as a dynamic forum where thought leaders dissect the nuances of Continual AI’s impact on both academia and industry.
Gaining Fresh Perspectives from Continual AI’s Blog on Medium
Continual AI’s blog on Medium serves as an intellectual hub, where deep dives into theoretical frameworks and practical applications coalesce. Beyond traditional platforms, this blog unveils fresh perspectives, providing a direct channel for thought leaders to share their insights. Whether it’s demystifying complex algorithms, exploring real-world implementations, or addressing industry trends, the Medium blog acts as a bridge between theoretical discussions and tangible applications. Readers gain access to the latest ruminations from thought leaders, fostering a dynamic dialogue that pushes the boundaries of what Continual AI can achieve.
This section aims to uncover the depth of Continual AI insights from thought leaders, traversing the dynamic landscape of the LinkedIn page and delving into the rich content offered by the Medium blog. Through these channels, readers gain access to diverse perspectives, further enriching their understanding of Continual AI’s multifaceted impact.
Engaging with the Continual AI Community
The Continual AI Unconference: An Interactive Forum for Knowledge Exchange
The heart of Continual AI’s community engagement beats vibrantly at the Continual AI Unconference—an avant-garde platform fostering interactive knowledge exchange. This unconference transcends traditional boundaries, providing a dynamic space where enthusiasts, researchers, and practitioners converge. Attendees actively shape the agenda, fostering discussions on the latest trends, challenges, and breakthroughs in Continual AI. The unconference embodies the spirit of continual learning, reflecting the very principles it champions. It’s not just an event; it’s a collaborative journey, where the community collectively propels the frontiers of Continual AI.
Exploring Continual AI’s Presence on YouTube for Insightful Content
The narrative of Continual AI unfolds vividly on YouTube, where the community converges for insightful content. From tutorials unraveling the intricacies of Avalanche Continual AI to discussions with industry experts, the platform serves as a visual gateway into the world of Continual AI. Exploring this digital realm offers a dynamic complement to traditional learning, providing a richer understanding of concepts through engaging visuals. YouTube becomes a space where the community not only consumes but actively participates, creating a multimedia tapestry that encapsulates the evolving narrative of Continual AI.
In this exploration, we immerse ourselves in the interactive realm of the Continual AI Unconference and venture into the visually compelling domain of YouTube, uncovering how these platforms become epicenters for community engagement, knowledge exchange, and a dynamic showcase of Continual AI’s real-world impact.
Making Your AI More Efficient with Continual Learning
Practical Strategies for Integrating Continual Learning into AI Models
The journey towards making AI more efficient unfolds through the practical integration of Continual Learning strategies. This section delves into actionable approaches for seamlessly incorporating Continual Learning into AI models. From designing adaptable architectures to implementing efficient data management practices, these strategies provide a roadmap for developers and AI practitioners. It’s not merely a theoretical concept but a hands-on guide to infuse the principles of Continual AI into the very fabric of machine learning models.
Addressing Challenges and Optimizing Efficiency
As with any transformative paradigm, challenges arise on the path to efficiency. Here, we navigate through the hurdles and pitfalls associated with adopting Continual Learning in AI models. Addressing the notorious “catastrophic forgetting” problem becomes paramount, and strategies to mitigate this challenge take center stage. Moreover, optimizing efficiency involves a nuanced understanding of model deployment, fine-tuning, and continuous monitoring. This section unveils a comprehensive approach to overcoming challenges and fine-tuning AI models for maximum efficiency.
In this segment, the focus shifts from theoretical concepts to actionable steps for practitioners looking to enhance the efficiency of their AI models. From hands-on strategies to tackle challenges to optimizing overall efficiency, this exploration provides practical insights for those navigating the Continual AI landscape.
Conclusion
In a world where adaptability is paramount, Continual AI emerges not just as a technology but as a transformative force, a beacon of innovation illuminating the path to a dynamic future in machine learning. As we navigate through the layers of Continual AI, from its theoretical underpinnings to its tangible applications, a profound understanding unfolds. It goes beyond being a mere concept; it’s a paradigm that reshapes the landscape of artificial intelligence.
This blog serves as a guide through the expansive realms of Continual AI, providing readers with insights into its applications that stretch from Avalanche Continual AI’s groundbreaking contributions to the dynamic GitHub repository. The career prospects within this evolving landscape are illuminated, emphasizing the skills and expertise that set professionals on the path to success.
Our journey doesn’t end with applications and careers; it extends into the heart of research insights, where Continual Learning becomes a catalyst for AI improvement. Thought leaders on platforms like LinkedIn and Medium offer fresh perspectives, enriching our understanding of Continual AI’s multifaceted impact. Engaging with the community through unconferences and YouTube amplifies the narrative, transforming this technology into a living, breathing entity shaped by collective knowledge.
As we conclude, the transformative impact of Continual AI is undeniable. It’s not just a tool; it’s a continuous evolution, an ever-adapting intelligence that mirrors the dynamism of the world it navigates. In the continuum of machine learning, Continual AI stands tall, inviting us to embrace a future where adaptability and continuous learning aren’t just principles but the driving force behind the next wave of innovation.